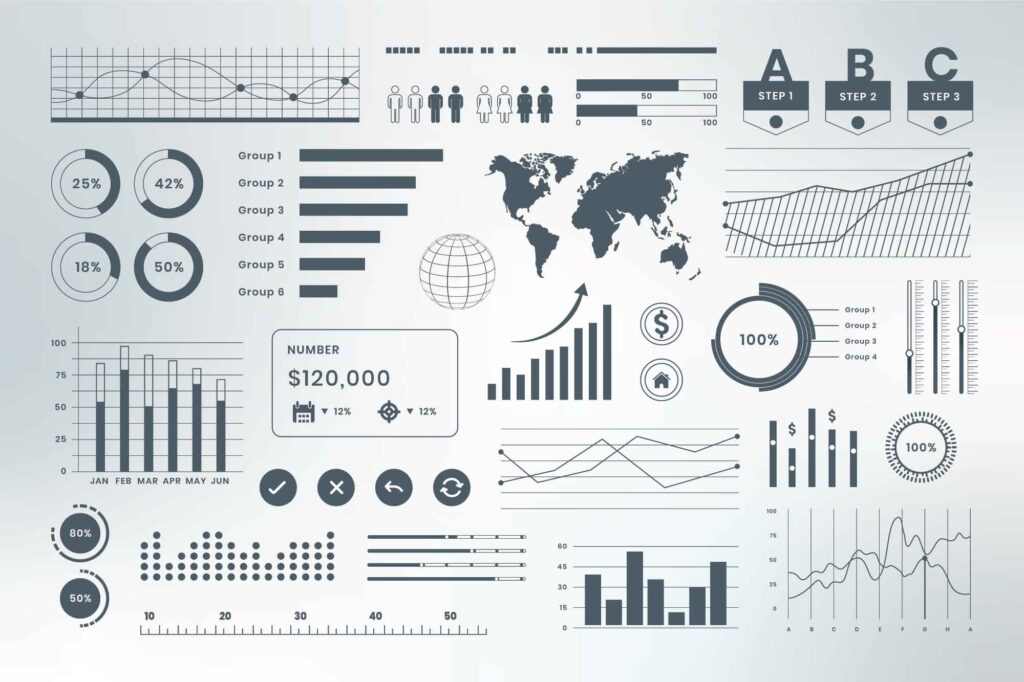
How to pull insights from data.
Many managers are in charge of discovering process improvements. They often have a list that intuitively feels right to move forward with or investigate further. Some common examples being maintenance scheduling or customer service response time. If you’re a service company, you might be interested in closed ticket rate, time entry, or maybe it’s even predicting sales for the next quarter. Many organizations find conflict understanding what data to look at how to qualify and quantify an idea that might come from reviewing data. There may be concerns with resources with the necessary skills or people throughout the company that actually have the time, interest, and motivation to see these projects through.
What we find helpful is to truly understand what data can do, understand what data is, and identify worthy problems to solve. This article intends to guide you through understanding data, how to qualify if a data project or idea is worth the time and effort to proceed. We also want to answer questions about resources and how to fit technology innovation within an overall strategy.
Data has never been easier to collect and store. Anytime you interact with the Internet or an Internet of Things device (smartphones, watches, GPS, etc.), data like location, clicks, text, time, etc., is collected and stored. Data can be tracked through company ERP systems CRM systems, machines connected to the Internet like CNC machines, mobile devices, Smart Tools, and anything connected to the Internet can be easily tracked and stored. Typically data is stored in servers that can be local to your office or cloud with a company like Microsoft or Amazon. These cloud servers are still physical but often far from where you operate the business. This tracked data is typically represented in tables like excel documents, images like actual pictures, or unstructured, which is just a hard code like HTML code.
What to consider with data.
Data is objective; it is hard, cold, and factual. Where things can go wrong is in the interpretation of data. Assumptions and pitfalls with data can influence the insight or result. Things to consider are the data source, who is behind the creation or generation of data, who holds the evidence, and what motivations the people want to collect or store the data. Are they making any poor correlations or assumptions with the data? This is by no means an exhaustive list of how data can be skewed but rather a limited set. The message here is that when analyzing data, you need to be conscious of possible bias. For example, a motivated employee wants to solve an issue they deal with daily, like time entry. Their motivations may be well-intended, but the communication about the data or how they present the effects can be altered. This may happen because of how close they are to the problem and how it directly impacts them. Something else to consider is the sample size of the data. The larger the sample size and the larger the data set, the higher the quality.
We find many business owners or other stakeholders who are curious about what artificial intelligence is, what machine learning is and how to use it within their business. Artificial intelligence, broadly defined, is a machine or computer system that mimics human intelligence. It can be something as simple as an automated receptionist when someone calls into your business. Or it could be as complex as facial and voice recognition. Machine learning is a subset of artificial intelligence. It reduces the reliance on human intervention in predicting outcomes by reviewing data. Machine learning uses statistical models to make predictions. Similar to the example that when you flip a coin, it’s 50% odds of tails 50% odds of heads over a given period. You can build statistical models to identify different facial features, different colours, different animals, or different product types. It is using statistical models to remove the need for humans to manually make those identifications. You may have heard of deep learning. Deep learning is another subset of artificial intelligence where predictive models are based on how the human mind works using neural networks. Deep learning aims to reduce the need for human intervention for prediction and decision-making with the use of data.
What is data good and not good for?
It’s beneficial to understand what data is good and not suitable for. What data is good for is discovering a problem. What is not suitable is identifying why something is happening. In the rush for companies to be more objective-based and data-driven, there is still a place for traditional qualitative research and analysis. Undergoing workshops and case studies are essential in making fully informed decisions regarding problems in your business and its solutions. Data will tell you what the problem is, but it will not tell you the answer. For example, data is great at telling you what demographics of customers buy what products like what generations of consumers prefer what types of food. But data cannot tell you why they buy—understanding the why is the place for qualitative research. Asking people why they made their decisions, their motivations, and what influenced them will aid your data. This helps in making clear decisions to solve problems or create solutions.
So how do we put this all together? When evaluating an idea or trying to solve a business problem, look for opportunities with a substantial amount of data. Understand how to collect that data and where it is stored. If you think there is a problem to solve, but there isn’t any data collection in place, it might not be worth cashing until data collection is in place. When reviewing or having a data analyst review it, be aware of the assumptions and the biases that the people collecting the data may have. If you’re interested in using artificial intelligence machine learning or deep learning, learn what those technologies are and how predictive models can provide you with information that will improve your targeted process.
How to work with data.
We recommend clearly identifying the problem you’re trying to solve. Avoid terminology like improved production. Instead, determine a clear KPI and a measurement associated with the overall goal. For example, decreasing product shipping time by 20% in the next two quarters. If you’re not already tracking or don’t have a tool to track shipping time, you should implement one before proceeding. We find the use of deadlines effective in creating solutions to problems. Otherwise, day-to-day issues or other priorities may take precedence. Give a shorter timeline than you may think you need; it’s easy to push projects like this for years. You must understand and acknowledge the limits of the team related to the business problem. We have found that working systematically and one problem at a time is the approach for best success. One of the critical determinants of project success is having executive or ownership buy-in; the confidence that this delivers to the team actively working on the project helps support and continue their motivation to see the project through to its end. We have found that innovation is the best spread around and not just kept internally. The use of third-party support helps to accelerate the success of projects. No one can be a master of all domains, so having a third-party company that you can rely on for skill sets not in can drive your project forward. Having a connection with a third party or independent resource builds a community of innovation. Outside individuals have less bias and less proximity to the problem, making the overall process more objective. Finally, having access to domain experts will limit assumptions and decrease errors. Domain experts can be actual employees who work day-to-day within the problem you’re trying to solve or specialized people inside or outside of the organization with the missing skills from your project team.
What we do at solut is help organizations through this process by identifying and qualifying problems or ideas. We do this through assessments, workshops, and audits. We try and act that inform the third party to create a community of innovation and deliver the skills that may be gaps at your organization. We see ourselves as experts in data analytics, visualization, and building applications to streamline bottlenecks, increasing performance and business processes. If you are curious about what problems can be solved with your organization’s data or need help solving issues already identified, we are here for you.